McKinsey: Six ways to solve the analytics adoption challenge
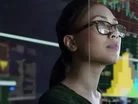
Mining companies have a huge untapped potential when it comes to analytics, with relatively low rates of adoption industry-wide creating openings for committed leaders to gain a competitive advantage, a recent report from McKinsey & Company says.
Businesses that lay the right groundwork for analytics success – by building engagement and alignment and by making analytics easy to use and the value easy to track – can cultivate a mindset and a shift in processes that could sustain double-digit returns on their analytics invest, the report, entitled: ‘Engaging employees to use analytics: How mining companies solve the adoption challenge’, said.
However, the mining industry is extremely complex, with plants operating like small cities, with hundreds of people and thousands of pieces of equipment. Almost every process requires specialised expertise and careful choreography – metallurgists have to adjust procedures for site conditions, mine engineers must continually tailor mine plans, and so on.
Knowing what measures to dial up on, or pull back on, is essential for the continued operation of a mine, but the complexities of the industry mean a slower adoption of analytics, the report says. This is primarily down to metallurgists, operators, and planners, and other stakeholders needing greater buy into the efforts.
This has proved difficult as many of these stakeholders hold highly specialised roles and there is considerable resistance to the idea that their expertise can be codified as an analytics tool, or that the initiatives will be managed by others with no operational context.
Despite this reticence, some large mining operations have begun deploying analytics at scale and building widespread adoption. One open-pit mining operation deployed real-time data on location and road conditions to increase material movement efficiency by five percent, while superior modelling helped a copper mine increase production 10 to 15 percent by improving throughput and recovery.
Therefore, from looking at 140 analytics implementations across multiple mining operations, the McKinsey & Company report found that engaging experts and frontline users, fostering ongoing collaboration, and maintaining a zealous focus on impact are keys to driving adoption and value.
From these studies, the report has outlined six ways that analytics leaders can drive adoption – not just in the mining industry, but across other sectors:
1. Build analytics products with domain experts and influencers
One of the most effective ways to engender trust is to get metallurgists, process leaders, and other subject experts directly involved in the development effort. Doing so allows experts to kick the tires of the emerging models and gain confidence in the analytics. Engaging them also enables higher-quality output, which helps to make sure the right data and variables are factored into the design in formats that users can understand and apply.
Expert engagement should also continue in the field as their close involvement will help to continuously inform and improve the analytical model, ensuring that the underlying logic is sound and the outputs actionable.
Including these experts also has another benefit – as they become invested in the success of a project, they will help other stakeholders to gain trust in it as well.
2. Establish a shared understanding at all levels
Analytics can mean different things to different people and organisations can avoid disconnects by creating a shared understanding of the program’s top-line objectives and appointing a product owner with the leadership clout and managerial skills to drive the initiative. The product owner and her or his team should strive to make the inner workings transparent and explainable.
Data governance also is crucial. Data must be well organised and accessible, with core dictionaries, terms, and formulas standardised. Leaders, designers, and users need to know the major sources of data being used and the way the algorithm’s recommendations are generated, this knowledge drives trust and acceptance of the model at the frontline level.
Communication is essential to the success of analytics initiatives. A clear messaging and awareness-building campaign establishes support, and tailored training programs equip employees to succeed.
3. Integrate analytics into existing workflow processes
The more familiar and intuitive a model is, the more likely it is to gain acceptance. Tailoring the interface to the specific user context, configuring steps to mirror the ones users would normally take to complete a particular task, and integrating the analytics into core processes can make new systems feel like a natural extension of existing ones, rather than an abrupt change.
Analytics teams also need to make sure that any new system is designed to integrate smoothly with the mine’s back-end technology stack. Otherwise, companies can end up with stand-alone models that eventually fall out of use because they cannot be updated or scaled.
4. Employ agile techniques to foster ownership and empowerment
Decision makers far removed from the field invest in prebuilt tools with a specific set of goals in mind, and they pass along instructions for local teams to use in prescribed ways. This approach can work well for enterprise resource planning (ERP) and other applications that handle routine back-office processes, but analytics initiatives typically involve frontline workers and managers. When field- and plant-based employees get directions from afar, they often feel that management devalues their expertise.
One of the most effective ways to increase adoption is to engage users directly in the development process by employing agile techniques that give users more agency. Agile ways of working democratise ownership. This open, non-hierarchical approach creates a healthy problem-solving dynamic in which individuals can more easily and freely contribute insights to the solution, regardless of their seniority and experience. The result is greater innovation and agility.
5. Unlock value through holistic performance management
For analytics initiatives to gain traction, mining leaders need to connect the dots between the overall business objectives for the analytics program and the specific milestones and criteria that define success at different stages. Otherwise, maintenance may have one set of measures, plant and mine managers another, and executive sponsors still others, resulting in divergent expectations.
Without a cohesive understanding of value, clear accountability, and concrete prioritization of tasks, adoption and impact can suffer. Teams can have a hard time maintaining enthusiasm for an initiative, and leaders can have a hard time justifying continued investment.
To create a clear line of sight to value, project leaders need to create a management infrastructure that eliminates silos, aligns performance metrics across teams, and assigns ownership for delivering on them. Working backward from top-line goals, project leads within the design team should define individualized performance measures for each role and work stream and ensure team members understand what is expected of them. Individuals and teams tasked with meeting specific goals can then prioritize workflow more effectively.
6. Anticipate the skills needed to scale and sustain
For mining organisations that are just beginning their analytics journeys, it can be easy to focus on the use cases, tools, and algorithms, since the learning curve for each can be steep. However, transitioning from pilot to production requires planning for scale from the start and building an in-house skill base capable of supporting the analytics portfolio over the long term.
Having the right expertise to maintain and train systems is one of the most important success factors with analytics. Model accuracy naturally drifts over time as operating conditions change. To account for that, businesses need to retrain the system continually—that is, roughly every three to six months. Otherwise, the quality of recommendations will drop, leading to fewer users and declining impact.